Patent Data for AI Patent Analytics
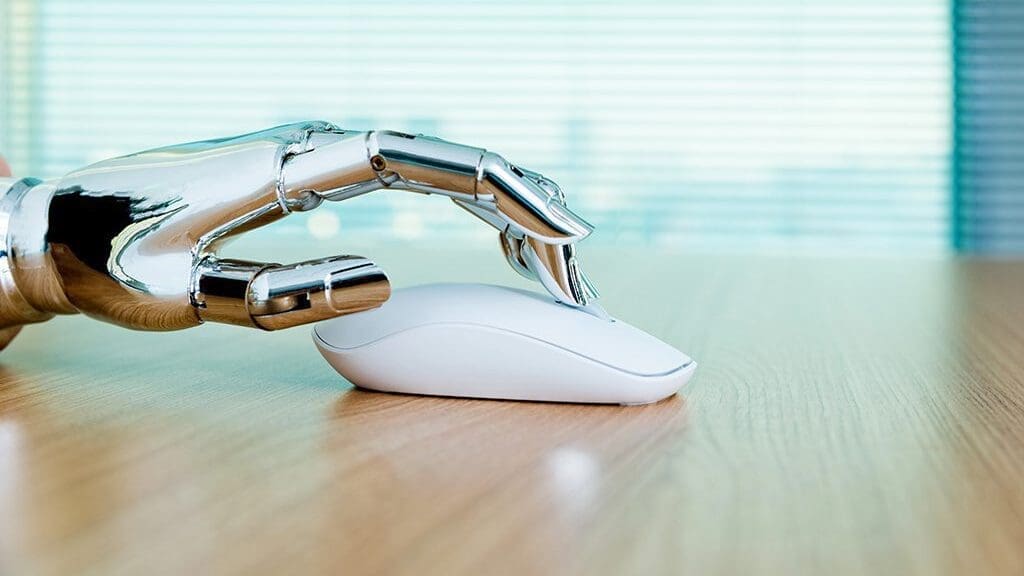
AI Patent Analytics Solutions Are Inevitable
Garbage In, Garbage Out – The Crucial Role of Data Quality
“Generative AI is the most powerful tool for creativity that has ever been created. It has the potential to unleash a new era of human innovation.” Elon Musk
AI patent analytics solutions are inevitable. It is easy to understand the generative AI hype. A conversation with a computer. What could be easier or more obvious as the next stage of technological development? The problem with hype, as the word suggests, is that it typically raises expectations beyond what is delivered or experienced. This tends to be followed by the ‘Trough of Disillusionment’ using the terminology coined by Gartner.
To help mitigate the potentially nauseous feelings generated by this AI rollercoaster, it is important to understand the fundamental building blocks that underlie the successful development and deployment of AI systems within the IP profession. This starts with data and data quality.
The importance of training data in the development of AI patent analytics tool
All applications that harness AI depend on the quality of input data. While it is obvious that poor input data will inevitably lead to poor output, what is harder is applying this basic rule to the plethora of AI solutions that are now readily available. If we start with the common example of machine translation, you enter source text in one language and output target text in another. There are significant differences in the quality of the many different available algorithms. Some of these differences can be attributed to how (and when) the algorithm was trained, while others depend on the technology deployed. This article compares Microsoft and Google translation algorithms and highlights the many different dimensions of the comparison. The scorecard is long and situation-dependent; for example, do you need it to respond to speech, or do you need to customize it?
Before attempting to apply any of this to intellectual property, a word about AI technology. The development of AI dates back to Turing (1950) or before. It comes in many flavors, including unsupervised and supervised machine learning and now generative AI. Even if you focus only on GenAI, there are many Large Language Models (LLMs) to choose from, and they have fundamentally different capabilities. For example, in a Statista 2023 ranking, Claude 3 Opus (Anthropic) scored 60.1% for the ability to solve maths problems, while Gemini Pro (Google) scored 32.6% (and the improved Gemini 1.5 Pro scored 58.5%). None of that matters if the problem you are trying to solve does not include numbers.
Choosing the right AI patent analytics approach for your problem
So, when Elon Musk suggests that GenAI has the potential to unleash a new era of human innovation, what does that mean for the IP profession? The answer is many things. Innovators at the heart of an R&D team could accelerate a project or stimulate new lines of inquiry. Patent professionals could automate patent drafting or reduce the administrative burden of handling patent prosecution before a patent examiner.
For the last decade, LexisNexis Intellectual Property Solutions has been focused on a very specific set of strategic questions that go to the heart of the risk and value associated with patent rights. These include:
- the transition from quantity to patent quality
- competitive intelligence and benchmarking for disruptive technologies
- risk management in times of increased NPE activity and significant geopolitical changes
- evidencing investment in sustainable technologies using mapping of patented technology to UN SDGs. The recent WIPO Report on Mapping Innovations: Patents and the Sustainable Development Goals demonstrates the importance of this data
- facilitating evidence-based financial outcomes for Standard Essential Patents (SEP) licensing in telecommunication.
This overview does an injustice to the broad and diverse spectrum of IP opportunities. But here’s the challenge: It goes back to hype. Everyone is calling for more AI when what they are thinking is greater efficiency. For the IP professional, this means delivering solutions that are better, faster and cheaper (the so-called iron triangle) than the approaches adopted today. In the world of AI patent analytics, the triangle acquires new dimensions, such as trust and transparency.
Data quality
The answer to these many conundrums requires going back to basics. This can be illustrated by reference to patent analytics. Incredible though it sounds, the ability to digitally search for patents only started in 1998 (Delphion). By 2006 (the launch of Google Patents), patent data was ubiquitous and recognized as an essential source of scientific information. Today, there are dozens of proprietary patent data products, and many times that if you include the services offered by the national (e.g., USPTO) and international patent offices (e.g., Espacenet from EPO). Choosing the right source of patent analytics means focussing on what’s important:
- Accuracy – Patent data is messy, and while accessing public data from a national patent office might be free, it is often not clean. The most common problem here is ownership, where no attempt is made to group together patents owned by members of the same group. If you cannot attribute a patent to its current owner, all is lost.
- Completeness – An important aspect of this is the concept of a patent family, where patents filed in multiple jurisdictions relating to the same invention are treated as one invention. Another important aspect of this is global coverage.
- Accessibility – Patent analytics was originally only of interest to patent specialists involved in building patent portfolios. Today, demand is driven by non-IP teams, where speed and use become far more important.
Augmenting patent data with other value-added information
As patent data continues to grow in importance, it has become increasingly apparent that patent data is often not enough. Referring back to some of the strategic use cases for patent analytics:
- Quality – Many of the leading patent scoring algorithms, such as the Patent Asset Index, rely on both citation data and gross national income (GNI) data to adjust for the relative importance of patent rights in countries of different sizes
- Risk – When integrated and aligned, patent litigation data is a good indicator of patent risk.
- Technology – businesses think in terms of technology trends, and the ability to analyze patents through that lens is essential
- SEPs – Mappings to the relevant standards will enhance patent databases that include SEP information.
In summary, while AI is important, the starting point is WHY. If you know the problem you want to solve, this can be your guide to the data you need. In the field of patents, not all data is the same. Even when dealing with ostensibly identical datasets, focus on quality. If you engage with incomplete or inaccurate datasets, then AI cannot begin to help. In the world of patent analytics, “rubbish-in” only ever has one outcome. This is true whether you are reviewing the data manually or using the latest in GenAI.
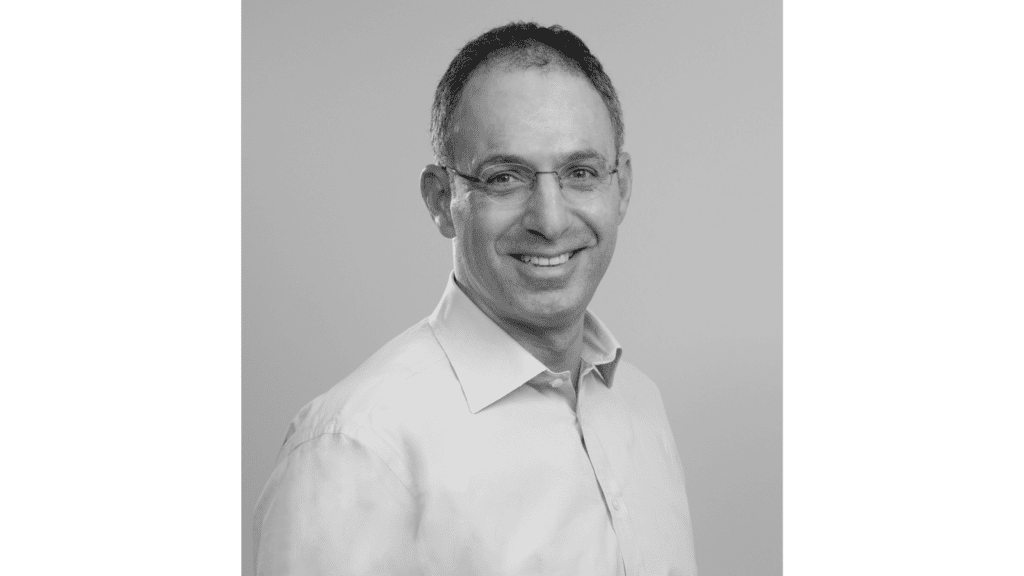
About Nigel
Nigel Swycher is co-founder and CEO of LexisNexis Cipher – His background is in in law, where he led the IP practice at leading law firm Slaughter and May.
For many years, the IAM 300 has recognized Nigel as a leader in IP strategy.
Join the AI-Insider program to shape the future of AI in IP Solutions
Members of the PatentSight+ AI-Insider program will lead the advancement of artificial intelligence in intellectual property and help revolutionize the way high-value strategic insights can be gained from
AI-powered IP search and analytics.
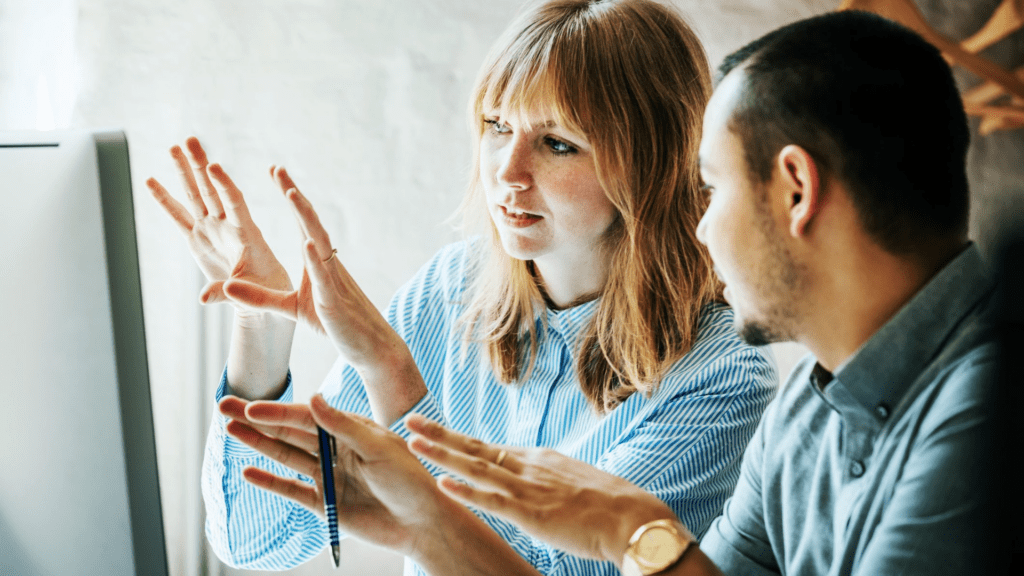
- Exclusive Access: Get early access to information on our latest AI products.
- Early insights: Shape the future of AI solutions by feedback in focus groups.
- Be on the Inside Track: Gain privileged access to resources, events, and updates.